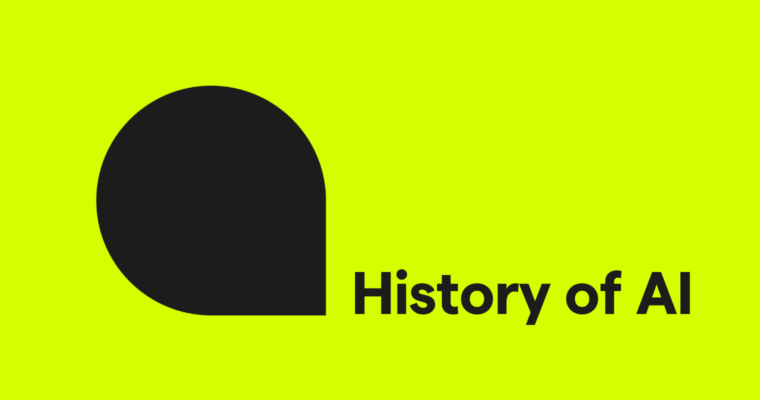
Artificial intelligence (AI) has evolved from science fiction and theoretical ideas into a fundamental part of contemporary technology and everyday life. Ideas that once inspired visionaries like Alan Turing have developed into smart systems that power industries, improve human abilities, and change how we engage with the world.
This article explores the key milestones that have shaped AI’s remarkable journey, highlighting the groundbreaking innovations and shifts in thought that have propelled it from its humble beginnings to its current state of transformative influence.
Table of contents
- What is AI?
- 1950s–1960s: Early achievements in AI
- 1970s: The first AI winter
- 1980s: A revival through expert systems
- 1980s–1990s: The second AI winter
- 1990s: Emergence of machine learning
- 2000s–2010s: The rise of deep learning
- 2020s: AI in the modern era
- Conclusion
What is AI?
Before exploring AI’s history, it’s important to first define what AI is and understand its fundamental capabilities.
At its core, AI refers to the ability of machines to mimic human intelligence, enabling them to learn from data, recognize patterns, make decisions, and solve problems. AI systems perform tasks that traditionally require human cognition, such as understanding natural language, recognizing images, and autonomously navigating environments.
By replicating aspects of human thought and reasoning, AI enhances efficiency, uncovers valuable insights, and addresses complex challenges across diverse fields. Understanding these foundational principles provides a key backdrop for exploring AI’s evolution—revealing the breakthroughs that transformed it from a conceptual vision into a revolutionary force shaping modern technology.
1950s–1960s: Early achievements in AI
The early years of AI were marked by groundbreaking innovations that laid the foundation for the field’s future. These advancements showcased AI’s potential and illuminated the challenges ahead.
- Alan Turing’s vision (1950): In his seminal paper “Computing Machinery and Intelligence,” Alan Turing asked, “Can machines think?” He introduced the Turing Test, a method to determine if a machine could mimic human conversation convincingly. This concept became a cornerstone of AI research.
- The birth of AI (1956): The Dartmouth Summer Research Project marked the official beginning of artificial intelligence as an academic field. During this pivotal conference, researchers coined the term “artificial intelligence” and initiated efforts to develop machines that could emulate human intelligence.
- Perceptron (1957): Frank Rosenblatt introduced the perceptron, an early neural network model capable of recognizing patterns. Although it was an important step toward machine learning, it had significant limitations, particularly in solving complex problems.
- Eliza (1966): Joseph Weizenbaum at MIT developed ELIZA, the first chatbot designed to simulate a psychotherapist. Utilizing natural language processing (NLP), ELIZA demonstrated the potential of conversational agents in AI and laid the foundation for future developments in human-computer interaction.
- Shakey the Robot (1966): Shakey was the first mobile robot capable of autonomous navigation and decision-making. It used sensors and logical reasoning to interact with its environment, showcasing the integration of perception, planning, and execution in robotics.
Key takeaways: The 1950s and 1960s were foundational years for AI, characterized by visionary ideas and innovative technologies that set the stage for future advancements.
1970s: The first AI winter
Despite early successes, the 1970s brought significant challenges that dampened the initial excitement around AI. This period, known as the “AI winter,” was marked by slowed progress and reduced funding.
- Criticism of neural networks (1969): In their book Perceptrons, researchers Marvin Minsky and Seymour Papert highlighted critical flaws in single-layer perceptrons, demonstrating their inability to solve certain complex problems. This criticism stalled neural network research for years, delaying progress in machine learning (ML).
- Funding cuts: Governments and companies reduced investments as AI failed to meet high expectations, leading to decreased enthusiasm and fewer advancements in AI research and development.
Key takeaway: The first AI winter underscored the importance of managing expectations and addressing the inherent challenges in AI development.
1980s: A revival through expert systems
AI made a strong comeback in the 1980s by focusing on practical solutions to real-world problems. This resurgence was driven by several key developments:
- Expert systems: Programs like MYCIN, designed to diagnose diseases, and XCON, used for configuring computer systems, demonstrated AI’s practical applications. These systems achieved commercial success in the 1980s, but their high cost, difficulty in scaling, and inability to handle uncertainty contributed to their decline by the late 1980s.
- Backpropagation (1986): Originally introduced by Paul Werbos in 1974, backpropagation gained prominence in 1986 when Rumelhart, Hinton, and Williams showcased its effectiveness in training multilayer neural networks. This breakthrough reignited interest in neural networks, setting the stage for deep learning advancements in later decades.
- Advancements in autonomous vehicles and NLP: Early prototypes of self-driving cars emerged from institutions like Carnegie Mellon University. Additionally, progress in NLP led to better speech recognition and machine translation, enhancing human-computer interactions.
Key takeaway: The 1980s demonstrated AI’s ability to solve specific, practical problems, leading to renewed investment and interest in the field.
1980s–1990s: The second AI winter
Despite progress in the early 1980s, the decade ended with another slowdown, leading to the “second AI winter.”
- High costs and limited power: Developing and running AI systems remained expensive and computationally intensive, making widespread adoption challenging.
- Overpromising and underdelivering: Unrealistic expectations led to disappointment as AI failed to deliver on lofty promises, resulting in reduced funding and skepticism.
Key takeaway: This period was less severe than the first AI winter, but it still slowed advancements. The second AI winter highlighted the need for realistic expectations and sustainable development practices in AI research.
1990s: Emergence of machine learning
The 1990s marked a pivotal shift toward machine learning, where computers learned patterns from data instead of following predefined rules. This era introduced several significant milestones:
- Support vector machines (SVMs): Originally developed by Vladimir Vapnik and Alexey Chervonenkis, SVMs gained significant adoption in the 1990s, particularly after the introduction of soft margin SVMs and the kernel trick. These advancements allowed SVMs to handle complex classification problems efficiently.
- Decision trees: Gained prominence as versatile and interpretable models for both classification and regression tasks. Their interpretability and ability to model complex decision-making processes made them essential tools in various applications. Additionally, decision trees laid the groundwork for ensemble methods, which further enhanced predictive performance.
- Ensemble techniques: Methods like bagging (1996) and boosting (1997) emerged, significantly improving prediction accuracy by aggregating multiple models. These techniques leveraged the strengths of individual algorithms to create more robust and reliable systems, forming the foundation of modern ensemble learning approaches.
- Real-world applications: AI was extensively applied in areas such as fraud detection, document classification, and facial recognition, demonstrating its practical utility across diverse industries.
- Reinforcement learning advancements: The 1990s saw important progress in reinforcement learning, particularly in the application of function approximation and policy iteration. Techniques like Q-learning, introduced in 1989, were refined and applied to more complex decision-making problems, paving the way for adaptive AI systems.
Key takeaways: The 1990s emphasized the practical value of machine learning, setting the stage for more ambitious and sophisticated AI applications in the future.
2000s–2010s: The rise of deep learning
The 2000s and 2010s marked a turning point in AI, driven by breakthroughs in deep learning. Advances in neural network architectures, training methods, and computational power led to rapid progress in AI capabilities. Key developments included:
- Deep Belief Networks (2006): Geoffrey Hinton and his team introduced a new way to train deep neural networks using unsupervised learning, overcoming challenges in deep model training and reigniting interest in AI.
- CNNs and AlexNet (2012): While convolutional neural networks (CNNs) were first developed in the late 1980s, they gained widespread adoption in 2012 with AlexNet. This breakthrough utilized GPU acceleration to train a deep network on the ImageNet dataset, achieving record-breaking performance and sparking a new era of deep learning.
- RNNs and LSTMs (2010s): Recurrent neural networks (RNNs), particularly long short-term memory networks (LSTMs), became the foundation for speech recognition, machine translation, and time-series prediction, improving AI’s ability to process sequential data.
- Transformer architecture (2017): In the paper “Attention Is All You Need,” Vaswani et al. introduced the transformer model, which revolutionized NLP by replacing RNNs. By utilizing self-attention mechanisms, transformers significantly improved efficiency and accuracy in language modeling, leading to major advancements in AI-powered text processing.
- Large language models (2018): AI saw a paradigm shift with BERT (developed by Google in 2018) and GPT (developed by OpenAI in 2018), which transformed NLP by enabling machines to understand and generate human-like text, powering applications in chatbots, search engines, and content generation.
Key takeaway: Deep learning drove AI’s rapid evolution, unlocking new possibilities in image recognition, speech processing, and natural language understanding. These breakthroughs laid the foundation for the powerful AI systems we use today.
2020s: AI in the modern era
Today, AI is deeply embedded in daily life, shaping industries, automating tasks, and enhancing human capabilities. From virtual assistants and recommendation systems to autonomous vehicles and advanced medical diagnostics, AI has become a driving force behind technological innovation. The 2020s have witnessed a rapid acceleration in AI capabilities, marked by several groundbreaking developments that are reshaping how we work, create, and interact.
LLMs: Transforming AI
LLMs have emerged as a cornerstone of modern AI, trained on massive datasets to understand, generate, and refine human-like text with remarkable accuracy. These models, powered by deep learning architectures such as transformers, have revolutionized multiple domains, including communication, research, and content creation.
Key capabilities and impact:
- Context-aware text generation: LLMs produce coherent, contextually relevant text across a variety of applications, from drafting emails to summarizing research papers.
- Writing, coding, and creativity: They assist users in generating high-quality content, composing code, and even crafting poetry, novels, and scripts. Models like GitHub Copilot have redefined programming efficiency, enabling AI-assisted software development.
- Conversational AI: LLM-powered chatbots and virtual assistants provide human-like interaction in customer service, education, and healthcare, making information more accessible.
By enhancing communication, automating knowledge work, and enabling more intuitive human-AI interactions, LLMs are not only optimizing productivity but also paving the way for more advanced AI systems that can understand and reason like humans.
Generative AI: Unlocking creativity
Generative AI marks a transformative leap in how machines contribute to creative processes, enabling the production of original content across various domains. Unlike traditional AI, generative systems focus on creating new outputs rather than analyzing or solving predefined problems. Key areas of impact include:
- Text generation: Tools like Grammarly, ChatGPT, and Gemini streamline communication by generating human-like dialogue, articles, and reports from simple prompts, enhancing productivity and creativity.
- Image creation: Platforms like OpenAI’s DALL-E turn textual descriptions into custom, high-quality visuals, revolutionizing design, advertising, and the visual arts.
- Music and video production: AI systems can compose music, produce videos, and support creators in pushing the boundaries of art and storytelling, democratizing access to professional-grade tools.
These advancements enable personalized and scalable content creation at unprecedented levels, redefining creativity across industries. Generative AI has become not just a tool for problem-solving but a collaborative force, empowering creators to work faster, innovate boldly, and engage more deeply with their audiences. Its potential to reshape how humans and machines co-create continues to grow with each breakthrough.
Future prospects: AGI and ASI
While today’s AI systems excel in specialized tasks (narrow AI), researchers are making significant strides toward artificial general intelligence (AGI)—a level of AI capable of performing any intellectual task a human can. Achieving AGI would mark a major transition from task-specific models to systems with autonomous reasoning, learning, and adaptation across multiple domains, fundamentally reshaping technology’s role in society.
Beyond AGI, artificial superintelligence (ASI) represents a theoretical stage where AI surpasses human intelligence across all fields. The potential benefits of ASI are vast, from solving complex scientific challenges to revolutionizing medical research and innovation. However, its development introduces profound ethical, existential, and safety considerations, requiring proactive governance, alignment with human values, and robust safeguards to ensure responsible deployment.
Key takeaway: The 2020s have solidified AI’s role as an indispensable part of modern life, fueling advancements in automation, creativity, and problem-solving. With LLMs transforming communication, generative AI redefining creativity, and AGI research progressing, the decade has laid the foundation for a future where AI is not just a tool but an active collaborator in shaping human progress.
As AI continues evolving, the choices we make today regarding its development, governance, and ethical considerations will determine whether it becomes a force for innovation, empowerment, and global betterment—or a challenge to be reckoned with.
Conclusion
From Alan Turing’s foundational questions to today’s breakthroughs in deep learning and generative AI, the history of artificial intelligence is one of relentless innovation and transformation. Once a theoretical pursuit, AI now shapes industries, enhances human capabilities, and redefines creativity and problem-solving.
Looking ahead, AI’s evolution will push toward AGI, promising systems that reason, learn, and adapt across domains. Yet these advancements bring ethical and societal challenges, making responsible governance crucial. The future of AI will not just be about technological progress but about ensuring it serves humanity’s best interests. If guided wisely, AI can amplify human potential, drive discovery, and address some of our greatest challenges—shaping the course of the 21st century and beyond.