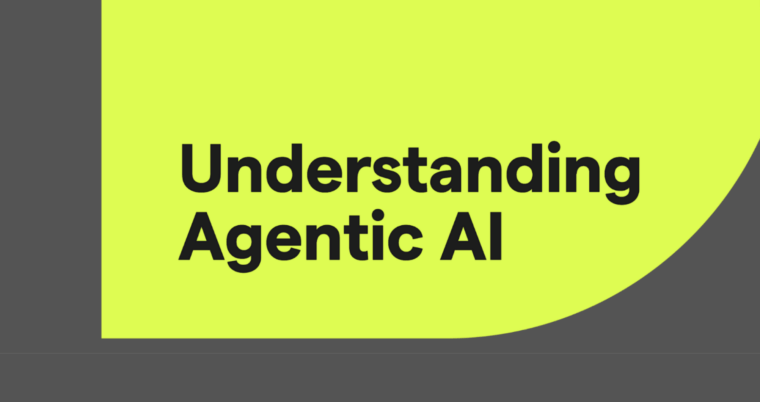
As artificial intelligence (AI) continues to evolve, a new category is emerging: agentic systems. These systems are capable of making decisions and acting independently of humans in changing environments. This powerful process, where the agentic system optimizes its actions over time based on changing external conditions and feedback from previous outcomes, is called sense-think-act. With applications such as improved customer service at scale, intelligent supply chain operations, and much in between, agentic systems are helping companies of all sizes improve business efficiency, reduce costs without sacrificing quality, and increase competitive advantage.
Table of contents
- What is agentic AI?
- How does agentic AI work?
- Agentic AI vs. generative and traditional AI
- Applications of agentic AI
- Benefits of agentic AI
- Challenges of agentic AI
- Conclusion
What is agentic AI?
Agentic AI describes a category of AI systems designed to take independent action in the real world based on their understanding of an environment. Unlike traditional AI systems, which provide feedback and analysis for use by humans, agentic AI independently evaluates the state of its environment, sets goals, and executes the actual actions in the real world that are necessary to achieve those goals. This ability to act autonomously is a consequence of agentic AI systems’ ability to choose a course of action without referencing predefined rules at every step. They are, therefore, adaptable and show context-aware behavior in complex, real-world situations.
For example, in manufacturing, agentic systems can monitor equipment performance, demand fluctuations, and other relevant factors in real time. They can then use this information to adjust equipment configuration, manage pipelines, and optimize production schedules without human oversight. They automatically adjust output and maintenance schedules in response to changing conditions.
How does agentic AI work?
Agentic AI continuously ingests and interprets data from its environment, learns patterns from that information, and makes decisions accordingly to achieve specific objectives. For enterprises, agentic AI can help continuously optimize many business operations, such as supply chain logistics and customer service pipelines, without human intervention. This process, called sense-think-act, is how the agentic system optimizes its actions over time based on changes in external conditions and feedback from previous outcomes:
- Sense: In this phase, the AI receives data inputs from the environment. “Sensations” can include structured business data like ERP systems or sales statistics, operational metrics from IoT sensors, unstructured data like customer communications, and performance feedback from previous actions.
- Think: Based on this sensed information, agentic AI uses decision-making frameworks to evaluate different possible actions. At this stage, the AI can weigh risks, predict outcomes, and choose the best course of action. Depending on the goals of the system and the environment in which it’s operating, agentic AI can use different “thinking” approaches, including reinforcement learning, deep learning, unsupervised learning, and transfer learning. For example, reinforcement learning can be used to optimize agent decisions through trial and error, while deep learning can help the agent find complex patterns in large datasets.
- Act: Once the right course of action is chosen, the AI changes its environment. This can involve responding to customer service inquiries or adjusting a manufacturing control system.
By monitoring results and environmental changes over time, the agent improves its decision-making process, allowing it to adapt strategies to changing conditions.
Agentic AI vs. generative and traditional AI: key differences
While agentic AI, traditional AI, and generative AI are all AI systems, they have some key differences. Understanding how these systems differ is critical for businesses when choosing the right AI solution based on their specific use cases and needs. The main differences are core function, level of autonomy, learning style, output, ability to handle complex tasks, and adaptability.
Agentic AI | Generative AI | Traditional AI | |
Core function | Autonomous decision-making and actions | Generates data or content based on input | Follows predefined rules |
Autonomy | High; capable of independent decision-making | Medium; creates output but cannot act independently | Low; reliant on predefined rules and human oversight |
Learning style | Adaptive, learns from the environment and adjusts behavior | Learns patterns but is constrained to its task scope | Limited flexibility; requires reprogramming for new tasks |
Output | Actions or decisions in response to real-world environments | New content, such as text or images | Solutions to specific, well-defined problems |
Ability to handle complex tasks | Handles unpredictable, complex tasks with changing variables | Performs creative tasks like content generation | Limited to structured, well-defined tasks |
Adaptability | Highly adaptive, learning in real time from new data. Can learn how to change its environment in novel ways. | Can innovate and adapt well within a limited task scope | Struggles with unfamiliar situations |
Use cases | Dynamic business operations (e.g., supply chain optimization, intelligent customer service systems, automated financial trading) | Creative content creation (e.g., marketing copy, code generation, document summaries) | Business tasks with fixed rules and static conditions (e.g., basic data analysis, automated report) |
Functionality and autonomy
Traditional AI is designed to solve specific, well-defined problems based on a narrow scope of predefined rules. This limits its capabilities to automation of rote analysis and human workflows, and it may or may not depend on human feedback. Generative AI produces new content like text or code, based on patterns learned from training data. It can handle much more complex analysis than traditional AI and incorporate reactivity, but it cannot take independent actions.
Agentic AI goes beyond these approaches by incorporating the ability to make complex decisions and act autonomously. It evaluates its environment, sets goals, and takes action without needing constant human intervention or predefined instructions.
Adaptability and learning
Another key difference between these AI types is their adaptability and learning capabilities. Traditional AI is typically confined to narrow tasks and struggles with new situations that fall outside of its original programming. Generative AI, although more dynamic than traditional AI, cannot inherently adapt—it generates creative outputs but doesn’t adjust to changes in real-time environments.
Agentic AI, however, is highly adaptable and can continuously learn and adjust based on its surroundings. It applies methods like reinforcement learning to refine its decision-making processes based on real-time feedback, making it suitable for situations where conditions are constantly changing.
Application focus
The differences in adaptability and functionality lead to specific applications for each type of AI. Traditional AI is great for narrow, well-defined tasks, like personalized recommendations and email spam classification. Generative AI thrives in creative environments, like writing blog posts or refactoring computer code.
Agentic AI, with its high level of autonomy and adaptability, is ideal for unpredictable and ever-changing environments, such as autonomous vehicles navigating complex road conditions or digital assistants capable of acting on behalf of users with minimal oversight.
Applications of agentic AI
With its ability to optimize processes, improve efficiency, and reduce costs, agentic AI has a wide range of practical applications across multiple industries, from healthcare to finance:
Healthcare operations
Operational efficiency is foundational to high-quality patient care in healthcare. Agentic AI, with its ability to adapt and learn, can help healthcare providers streamline patient services without sacrificing quality or needing to hire more personnel. For example, agentic AI can optimize patient scheduling based on resource allocation or power clinical decision-support systems that learn from patient outcomes and help healthcare providers make the right decisions for their patients.
Customer service
In many industries, efficient customer service is critical to business success. AI agents can help businesses streamline and optimize their customer service pipeline with minimal guidance from humans. For example, an agentic AI system might automatically route customer service tickets based on current demand and past interactions, or resolve complex customer questions without human intervention.
Warehouse and supply chain operations
In warehouse and supply chain operations, agentic AI can help robots perform complex tasks like sorting and packaging. Using data like item location and shifts in demand, these agentic systems can function well without waiting for human guidance. This adaptability and autonomy are valuable in fast-paced warehouse and supply chain environments, helping to scale the human workforce and increase efficiency.
Financial services
Banking and investment firms can use agentic AI to improve all aspects of their operations. In an investment firm, for example, agentic AI can adapt to market conditions in real time and make trades based on this information. Banks can also use agentic AI in risk assessment systems that dynamically adjust lending criteria or fraud detection systems that intelligently adapt to new threat patterns.
Benefits of agentic AI
Agentic AI offers notable benefits to businesses, including improved operational excellence, reduced costs, and a unique competitive advantage.
Reduced costs
With its ability to accurately complete complex tasks without human guidance, agentic AI can provide substantial cost savings. By using agentic AI to automate routine processes, businesses can reduce costs while maintaining service quality and scaling operations. This automation of routine processes also allows businesses to reallocate humans to more strategic tasks.
Operational excellence
Agentic AI means that businesses can support 24/7 operations without human oversight or an increase in operational complexity, improving operational excellence. Unlike other AI systems, agentic AI maintains consistent quality while continuously improving and adapting based on current environmental conditions and past outcomes. This helps businesses accelerate decision-making and remove bottlenecks, leading to quicker and more reliable business operations.
Competitive advantage
Agentic AI provides businesses with a significant competitive advantage through reduced costs and improved operational excellence. Instead of hiring, reallocating, or retraining personnel, businesses can use agentic AI to take data-driven action at a massive scale. As agentic AI systems learn and improve, they have the potential to replace some human functions, helping businesses scale and compete.
Challenges of agentic AI
Despite its potential, agentic AI comes with several challenges, including ethical concerns and security risks:
Ethical concerns
The autonomous capabilities of agentic AI raise a host of ethical concerns, especially in situations where the system’s actions could cause harm. For example, determining who is at fault if an autonomous vehicle is involved in an accident creates complex ethical and legal challenges. In addition, because agentic AI can operate without human oversight, issues around trust and fairness can arise, making transparency and ethics major concerns for developers and regulators.
Security risks
As a result of the ability to operate autonomously, agentic AI introduces new security vulnerabilities. If an agentic system is compromised, the potential for harm increases due to its capacity to take independent actions. This high degree of risk makes cybersecurity a critical part of agentic AI development and deployment.
Workforce dynamics and skill reallocation
Agentic systems have the potential to replace some human tasks, but they also require organizations to rethink workforce allocation. Implementing agentic AI often requires specialized roles, such as overseeing AI operations and maintaining systems. As a result, businesses may need to hire new personnel or upskill their existing workforce. Balancing the benefits of agentic AI with workforce dynamics is a critical consideration for any business.
Conclusion
Agentic AI is an important leap forward in AI, moving beyond the traditional and generative approaches with systems able to make decisions and act independently. While agentic systems provide numerous benefits—from increased operational efficiency and competitive advantage to reduced costs without sacrificing scale or quality—they also introduce new challenges, including ethical, compliance, and security risks. As developers and researchers address these challenges and continue to improve these systems, agentic AI will become more integrated into everyday applications, in customer service pipelines, supply chain operations, and much in between. For business leaders looking to stay ahead in the business landscape, understanding and strategically implementing agentic AI is critical.